Description
We have proposed in this project a new image model that combines the non-local means and sparse coding approaches to image restoration into a unified framework where similar patches are decomposed using similar sparsity patterns.
Quantitative and qualitative experiments with images corrupted with synthetic or real noise have shown that the proposed algorithm outperforms the state of the art in image demosaicking and denoising tasks. Next on our agenda is to include non-uniform noise models in the reconstruction process, then adapt our approach to other challenging image manipulation problems in computational photography, including deblurring, inpainting, and texture synthesis in still images and video sequences.
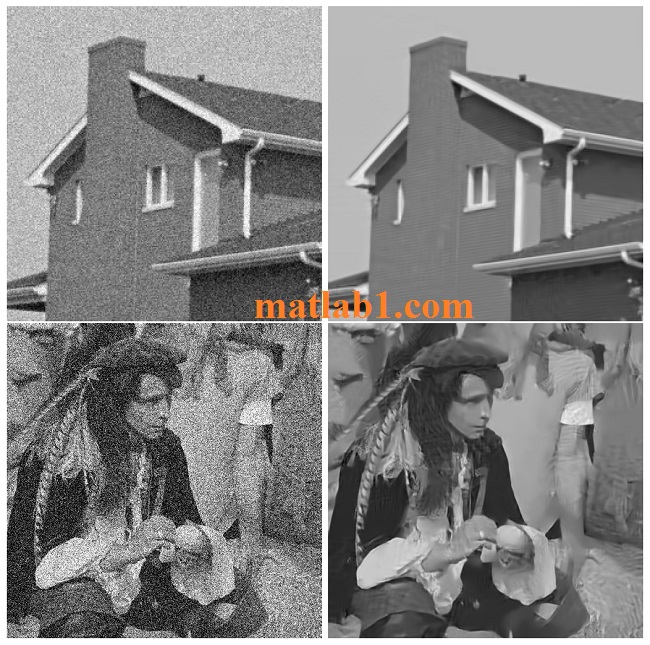
Qualitative evaluation of our denoising method with standard images. Left: noisy images. Right: restored images. Note that we reproduce the original brick texture in the house image (sigma = 15) and the hair texture for the man image (sigma = 50), both hardly visible in the noisy images. (The details are better seen by zooming on a computer screen.)
ref :
Mairal, Julien, Francis Bach, Jean Ponce, Guillermo Sapiro, and Andrew Zisserman. “Non-local sparse models for image restoration.” In Computer Vision, 2009 IEEE 12th International Conference on, pp. 2272-2279. IEEE, 2009.
Combination the non-local means and sparse coding approaches to image restoration
Reviews
There are no reviews yet.