Description
We developed an edge-guided LMMSE-type image interpolation technique. For each pixel to be interpolated, we partitioned its neighborhood into two observation subsets in two orthogonal directions. Each observation subset was used to generate an estimate of the missing sample. These two directional estimates were processed as two noisy measurements of the missing sample. Using and combining the statistics of the two observation subsets, we fused the two noisy measurements into a more robust estimate via linear minimum mean square-error estimation. To reduce the computational complexity of the proposed method, we simplified it to an optimal weighting problem and determined the optimal weights. The simplified method had competitive performance with significant computational savings. Experimental results showed that the presented methods avoided interpolation against edge directions and, hence, achieved noticeable reduction in ringing and other visual artifacts.
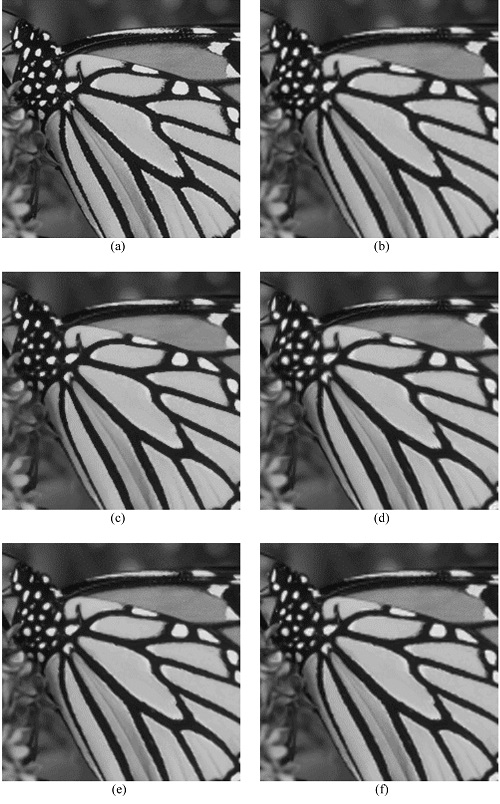
Interpolation results of the image Butterfly when the LR image is generated by low-pass filtering and downsampling the HR image (a) Original image,
interpolated image by (b) the cubic convolution, (c) the method in [8], (d) the method in [9], (e) the proposed LMMSE_INTR_cubic, and (f) the proposed
OW_INTR_cubic.
ref :
Zhang, Lei, and Xiaolin Wu. “An edge-guided image interpolation algorithm via directional filtering and data fusion.” IEEE transactions on Image Processing 15.8 (2006): 2226-2238.
Color Demosaicking by Local Directional Interpolation and Non-local Adaptive Thresholding
Nonlocally centralized sparse representation for image interpolation
.
Reviews
There are no reviews yet.