Description
What have we learned from the new theory of CSR and the above denoising experiments? It is enlightening to understand the relationship between dictionary learning and structural clustering from a manifold perspective. Globally the collection of patches in natural images would form a nonlinear manifold consisting of many constellations; how to discover the local geometry of such nonlinear manifold is a problem that has attracted lots of attention in recent years .
Image denoising can also be cast under the framework of manifold learning/reconstruction except that unsupervised learning works with noisy data. Dictionary learning such as K-SVD separates image signals from additive noise by thinking globally (i.e., change-of-coordinates); while structural clustering such as BM3D achieves the same objective by locally fitting the hypersurface in the patch space (i.e., iterative shrinkage). What CSR has shown is the benefit of combining global thinking with local fitting.
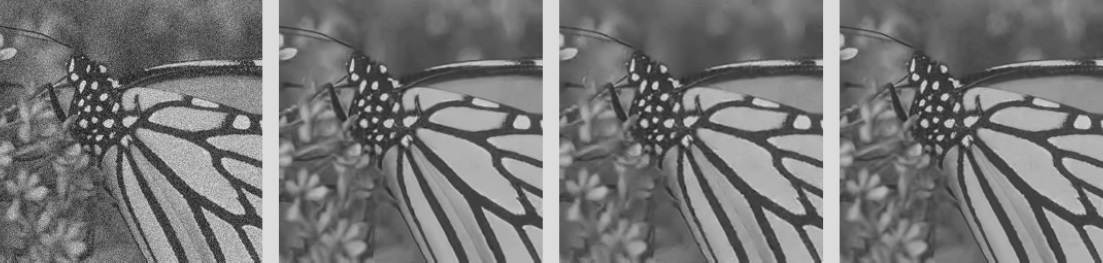
Denoising performance comparison for monarch image
ref :
Dong, W., Li, X., Zhang, L., & Shi, G. (2011, June). Sparsity-based image denoising via dictionary learning and structural clustering. In Computer Vision and Pattern Recognition (CVPR), 2011 IEEE Conference on (pp. 457-464). IEEE.
Image Denoising via Dictionary Learning and Structural Clustering
https://pdfs.semanticscholar.org/5872/de6fb6fd9969c26277a63d8ebb16431505bf.pdf
Reviews
There are no reviews yet.