Description
In this project, we implement a new image model named SSC–GSM that connects SSC with GSM and explore its applications into image restoration. The proposed SSC–GSM model attempts to characterize both the biased-mean (like inNCSR) and spatially-varying variance (like in GSM) of sparse coefficients.
It is shown that the formulated SSC–GSM problem, thanks to the power of alternating direction method of multipliers – can be decomposed into two subproblems both of which admit closed-form solutions when orthogonal basis is used. When applied to image restoration, SSC–GSM leads to computationally efficient algorithms involving iterative shrinkage/filtering only.
Extensive experimental results have shown that SSC–GSM can both preserve the sharpness of edges and suppress undesirable artifacts more effectively than other competing approaches. This work clearly shows the importance of spatial adaptation regardless the underlying image model is local or nonlocal; in fact, local variations and nonlocal invariance are two sides of the same coin – one has to take both of them into account during the art of image modeling.
In addition to image restoration, SSC–GSM can also be further studied along the line of dictionary learning. In our current implementation, we use PCA basis for its facilitating the derivation of analytical solutions. For non-unitary dictionary, we can solve the SSC–GSM problem by reducing it to iterative reweighted l1-minimization problem.
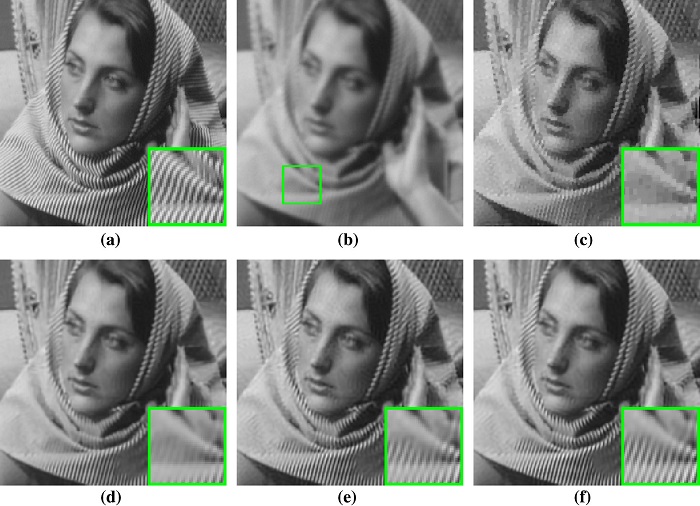
Deblurring performance comparison on the Barbara image.
(a) Original image; (b) Noisy and blurred image (Gaussian blur, σn = √ 2); deblurred images by (c) FISTA (Beck and Teboulle 2009) (PSNR=25.03 dB, SSIM=0.7377); (d) IDD-BM3D (Danielyan et al. 2012) (PSNR=27.19 dB, SSIM=0.8231); (e) NCSR (Dong et al. 2013b) (PSNR=27.91 dB, SSIM=0.8304); (f) Proposed SSC–GSM (PSNR=28.42 dB, SSIM=0.8462)

Image super-resolution performance comparison on the Plant
image (scaling factor 3, σn = 0). (a) Original image; (b) Lowresolution image; reconstructed images by (c) TV (Marquina and Osher 2008) (PSNR=31.34 dB, SSIM=0.8797); (d) Sparsity-based (Yang et al. 2010) (PSNR=31.55 dB, SSIM=0.8964); (e) NCSR (Dong et al. 2013b) (PSNR=34.00 dB, SSIM=0.9369); (f) Proposed SSC–GSM (PSNR=34.33 dB, SSIM=0.9236)
Train Bayesian neural network by Particle swarm optimization (PSO)
Zlata –
Awesome Work ! Very Professional.