Description
In this project , we proved that the BWH representation in [3] is equivalent to the usual target representation so that no new information can be introduced to improve the mean-shift tracking performance. We then proposed a CBWH method to reduce the relevance of background information and improve the target localisation. The proposed CBWH algorithm only transforms the histogram of target model and decreases the probability of target model features that are prominent in the background. The CBWH truly achieves what the BWH wants. The experimental results validated that CBWH can not only reduce the mean-shift iteration number but also improve the tracking accuracy. One of its important advantages is that it reduces the sensitivity of mean-shift tracking to the target initialisation so that CBWH can robustly track the target even if it is not well initialised.
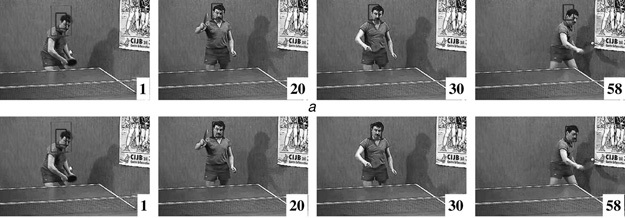
Mean-shift tracking results on the table tennis player sequence with another inaccurate initialisation Frames 1, 20, 30 and 58 are displayed
reference :
[3] Comaniciu, D., Ramesh, V., Meer, P.: ‘Kernel-based object tracking’, IEEE Trans. Pattern Anal. Mach. Intell., 2003, 25, (2), pp. 564–577
Reviews
There are no reviews yet.