Description
This paper revealed that it is the collaborative representation (CR) mechanism, but not the l1-norm sparsity constraint, that truly improves the face recognition (FR) accuracy. We then presented a very simple yet very effective FR scheme, namely CR based classification with regularized least square (CRC_RLS). Compared with the l1-regularized sparse representation based classification (SRC), the l2-regularized CRC_RLS has very competitive FR accuracy but with significantly lower complexity. The extensive experimental results clearly demonstrated that CRC_RLS is up to 1600 times faster than SRC without sacrificing recognition rate.
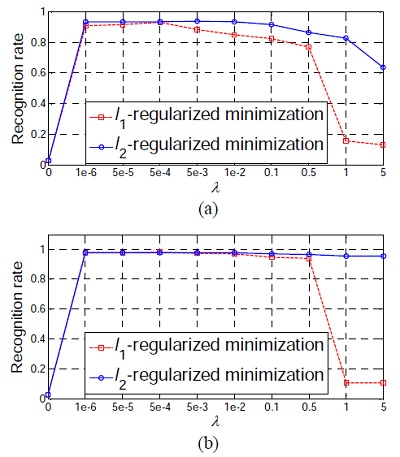
The recognition rates of SRC (l1-regularized
minimization) and CRC_RLS (l2-regularized minimization)
versus the different values of λ on the (a) AR and (b) Extended
Yale B databases;
reference :
Zhang, Lei, Meng Yang, and Xiangchu Feng. “Sparse representation or collaborative representation: Which helps face recognition?.” Computer vision (ICCV), 2011 IEEE international conference on. IEEE, 2011.
Nonlocally centralized sparse representation for image interpolation
https://en.wikipedia.org/wiki/Sparse_approximation
Reviews
There are no reviews yet.