Description
In this project, we proposed a Fisher Discrimination Dictionary Learning (FDDL) approach to sparse representation based image classification. The FDDL aims to learn a structured dictionary whose sub-dictionaries have specific class labels.
The discrimination ability of FDDL is two-folds. First, each sub-dictionary of the learned whole dictionary has good representation power to the samples from the corresponding class, but has poor representation power to the samples from other classes. Second, FDDL will result in discriminative coefficients by minimizing the with-class scatter and maximizing the between-class scatter of them.
Consequently, we presented the classification schemes associated with FDDL, which use both the discriminative reconstruction error and sparse coding coefficients to classify the input query image. The experimental results on face recognition (FR), digit recognition and gender classification clearly demonstrated the superiority of FDDL to many state-of-the-art dictionary learning based methods. In future, we will apply FDDL to other classification tasks such as object recognition.
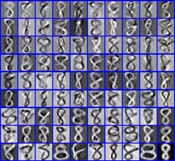
The learned bases of digits 8 by FDDL
ref :
Yang, Meng, et al. “Fisher discrimination dictionary learning for sparse representation.” Computer Vision (ICCV), 2011 IEEE International Conference on. IEEE, 2011.
Simplified Fisher Discrimination Dictionary Learning with application to Digit recognition
Reviews
There are no reviews yet.