practical way to monitor smoking and environmental factors
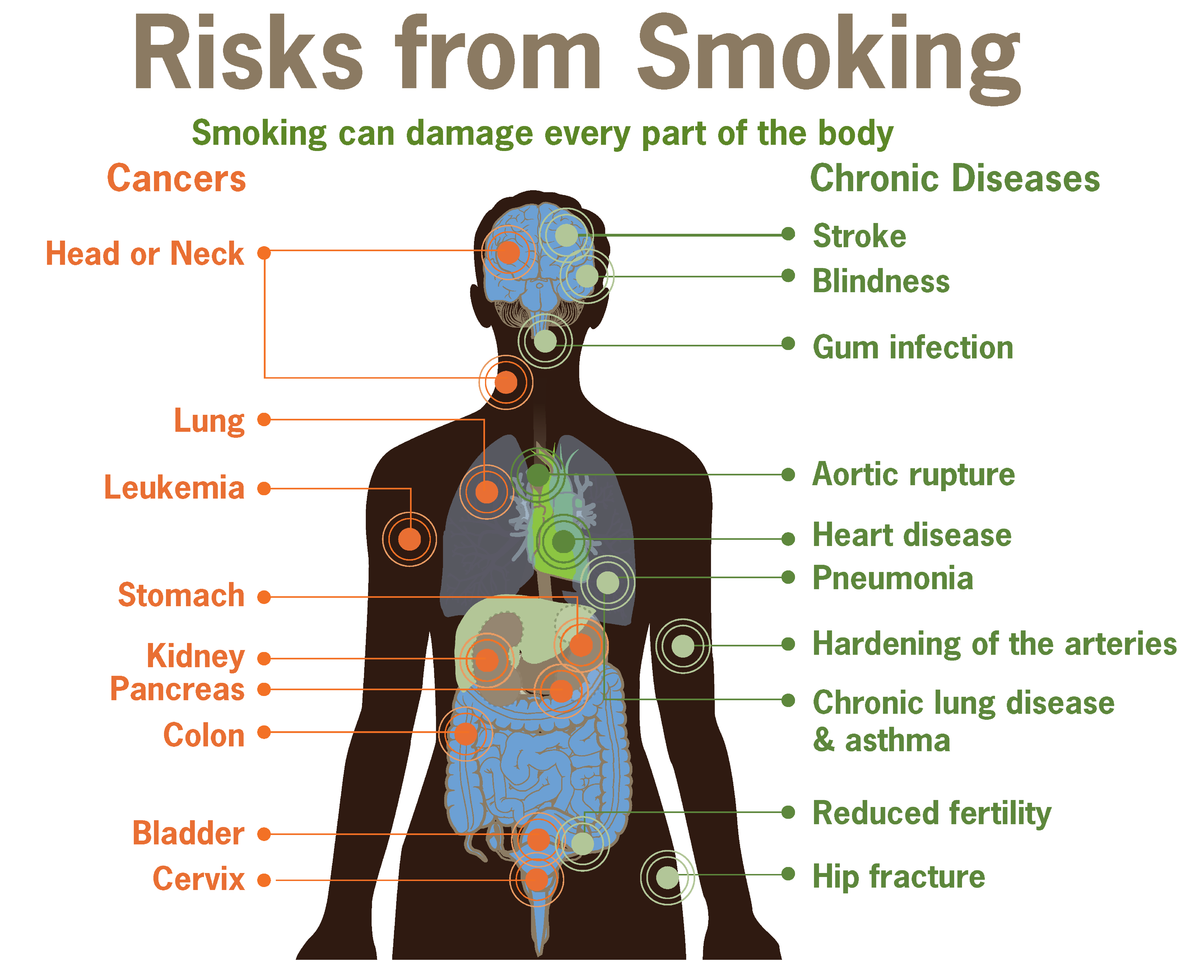
Risks form smoking
Cigarette smoking is the principal cause of premature death and disability in the United States. Tobacco smoking is causally linked to 13 different types of neoplastic disease. Of the 230,000 Americans who are killed by cancer every year, over two-thirds of the deaths are attributable to cigarette smoking. Smoking is also reported to be the cause of 6 million preventable deaths yearly. These statistics underscore the important role that smoking cessation research plays in reducing life-threatening diseases and death. Pervasive technologies create new health behavior measurement and intervention opportunities, which may also be applicable in tobacco addiction research and treatment. Smoking monitoring has been studied with technologies ranging from computer vision to text-messaging, but the widespread adoption of smartphones and the maturity of wearable sensing technology may provide an alternative, powerful, and practical way to monitor smoking and environmental factors that may influence smoking in daily life. For instance, computer vision systems are restricted to locations where cameras can be affordably installed and may raise privacy concerns; text messaging systems require user input and cannot proactively detect or intervene on behavior. Wearable technology may provide an affordable way to gather rich data about behavior, anywhere, and respond proactively to passively detected behavior, without creating privacy concerns. This paper describes an algorithm for automatic detection of puffs and smoking activity. Puffing is the handto- mouth gesture (HMG) while smoking. And smoking is a heterogeneous activity that may include multiple puffing, hand resting and tapping gestures. The algorithm uses a two-layer model, combining a supervised puffdetection classifier and a model based on common smoking topography, such as inter-puff interval and puff frequency. We explore detection of puffing and smoking from body-mounted motion sensors, where data are gathered from real smokers engaged in smoking and also other complex, similar activities, such as drinking, eating and using phone. To investigate the utility of various sensor positions, the datasets were collected with four accelerometers on the left and right wrists, dominant upper arm and dominant ankle. The smoking data includes examples of superposition, interleaving and ambiguity. Despite the complexity of the datasets relative to those used in prior work, the results outperform previously reported systems. Nevertheless, challenges were identified that researchers must ultimately resolve to support useful deployment of the algorithms in future applications. This work demonstrates why the smoking detection problem is deceptively complex.