introduction
With the explosion of online shopping sites, there has been a proliferation of businesses offering to post positive product reviews in exchange for payment, a practice known as \astroturng” . Many consumers rely on product reviews to make informed decisions about a purchase, and the presence of these deceptive reviews dilutes the candid customer feedback consumers expect with surreptitious advertising. Effective methods of detecting these deceptive reviews and/or products for which reviews have been purchased could enable companies to identify and crack down on reviewers and vendors engaging in these deceptive practices, improving customers’ online shopping experience. Recently, large online retailers such as Amazon.com have invested increasing resources in developing machine-learning solutions for combating fake reviews
In addition to the existence of companies that sell positive reviews, there appears to be a trend of sellers offering customers products for free or at reduced cost if they promise to post a review of the product. While many of the resulting reviews disclose that \[the customer] received this product in exchange for an honest review,” there is reason to believe that there exist additional reviews written under these conditions that do not include this disclaimer. While customers who include this disclaimer claim not to be biased by the receipt of a free product and their promise to post a review in exchange, we have reason to believe otherwise: 92.6% of the reviews we collected that contain this or any of a set of related statements are 4- or 5-star, while only 80.8% of all reviews collected are 4- or 5-star. The presence of these reviews further highlights the transformation of online review board into an advertising forum and supports the need for methods of detecting intentionally deceptive or otherwise
biased reviews.
Data
We collected over 1 million reviews for over 16,000 products from the online retail site Amazon.com between April and June of 2015. Reviews and product metadata were gathered using screen-scraping techniques. An initial script gathered URLs for the 5,000 top-listed products in each of 10 categories. While it is not clear how Amazon decides how to order their products, the top-listed products appeared to have more reviews on average. A subsequent script crawled the product pages for product metadata and all reviews. (The nal datasets contain fewer than 5,000 products because many products do not have any reviews, and some categories had fewer than 5,000 products.) We removed all products that did not have at least one review. For each of the 10 categories, we randomly selected 20% of the remaining products to be added to a held-out test set.
Feature Correlation Tests
In an eort to discover detectable textual and metadata features tending to correlate with fake reviews, we performed a number of correlation tests. For each test, we calculated the correlation between a pair of features using Pearson’s correlation coeffcient, given by the following equation:
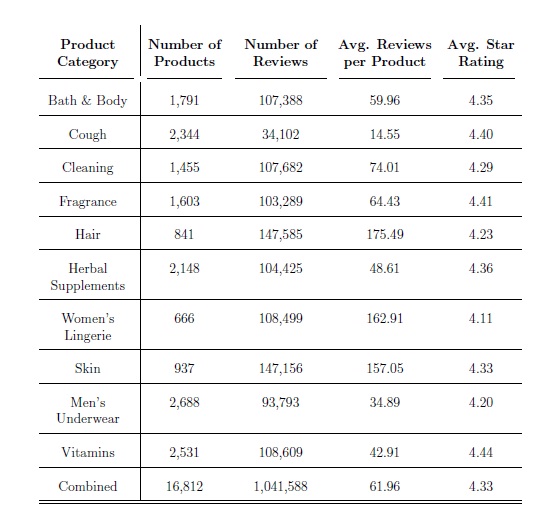
Table 3: Datasets Used for Correlation and Product Classication Experiments

Table 3.2: Data Used for Correlation and Product Classication Experiments: Training and Test Splits
Tests fell into two categories: those for which product-level feature values were used, andthose for which review-level feature values were used. Since we did not know which reviews were fake (or which products had a high incidence of fake reviews), each pair consisted of an indicator feature that we believed to correlate with fake reviews, which served as a rough measure of the review’s (or the product’s) \suspiciousness.” In the case of products, this was either the \star-rating skewedness,” a calculation of the percentage of reviews that were 5-star (or 5- and 1-star), or the variance of the reviews creations dates. In the case of reviews, this was either star-rating skewedness or the date on which the review was created. The choice of star-rating skewedness as an indicator feature was based on previous research suggesting that the star ratings of suspicious products tend to form a bimodal distribution . The choice of the variance of the creation dates of a products’ reviews was based on the hypothesis that products for whom a seller has purchased reviews tend to have a high density of reviews in a short period of time. Finally, the choice of the review creation date as an indicator feature was based on the hypothesis that the incidence of fake reviews was likely to increase over time.
Text-Based Product Classication
We explored the effectiveness of classifying products using various textual features of their 5-star reviews, using metadata features to generate the gold-standard class labels. The goal of these experiments was to determine whether the 5-star reviews of a suspicious product exhibited generalizable differences from the 5-star reviews of a typical product. All classication experiments were performed with MALLET using a maximum entropy classier and 4-fold cross-validation. For these experiments, products were labeled as \suspicious” or \trustworthy” based on the percentage of their reviews that were 5-star, or the percentage of their reviews that were 5- or 1-star. Products for which the percentage was high were considered to be suspicious, while products for which the percentage was low were considered to be trustworthy. For each experiment, a minimum threshold for this percentage was selected for suspicious products, and a maximum threshold was selected for trustworthy products. The thresholds were always set such that each class would have the same number of products. Because the gold-standard labels were based on the star rating distribution of products’ reviews, textual features were extracted from a product’s 5-star reviews only. This was done to prevent the model from classifying on the basis of the contrasting sentiment of reviews of different star ratings. If the classier learned to predict star ratings, it would be able to predict labels that had themselves been generated from distributions of star ratings with high accuracy, but it would not provide insight into our reasearch question. Furthermore, deceptive reviews are most likely to be 5-star or 1-star (depending on whether they are solicited by the seller or written by a competitor), and we were primarily interested in characterizing deceptive positive reviews. During the labeling step, we discarded products that did not have at least one 5-star review.
https://digital.lib.washington.edu/researchworks/handle/1773/33997