YouTube Algorithms
Algorithms – Pre 2012
The specifics of YouTube’s recommendation algorithm is a heavily-researched but tightly-kept secret. From the site’s inception, YouTube has been recommending videos based on the current content you are watching. On a rudimentary level, it has been shown that which videos are recommended come from specific things users can do when uploading videos. Tags, titles, and video description all contain keywords that both the YouTube search and the recommendation algorithm analyze when determining where the video “ranks” when compared to other videos with similar data. Research on virality of video content shows that there is a sort of “snowball” effect that YouTube puts into place once a video reaches a certain popularity (Pinto, Almeida, & Goncalves, 2013; Jiang, Miano, Yang, Lan, & Hauptmann, 2014; Gill, Arlitt, Li, & Mahanti, 2007).
From YouTube’s start in 2006 up to a key change in 2012, YouTube’s algorithm was most influenced by the “view count” of a video. View count simply counted when anyone clicked on a video in question, regardless of how much time the video was watched. Around this time, YouTube’s amateur content creation was split into two key categories: comedy sketches/highly scripted material, and vlogs (video blogs). The former consisted of channels like Smosh, which performed 5-10 minute comedy sketches, and JamesNintendoNerd2, also known as the Angry Video Game Nerd, who posted bi-monthly comedy skits revolving around video games (Burgess, Green, 2013). The latter had a wide range of young adult “vlogging:” users recording themselves talking about a particular topic for a length of time in an often unedited format (Snelson, 2015). During this time, “clickbaiting” or “viewbaiting” (Nalts, 2011) became an issue, as simply earning that first click was enough to bump you up in the rankings. Sexually provocative thumbnails or tagging your video with more popular (but incorrect) tags were common signs of those trying to abuse the system to their advantage. This provoked YouTube to make a change to its system, one that would cause a complete shift in content.
Algorithms – 2012 to the Present
In 2012, YouTube altered the recommendation algorithm in response to the influx of view-baiting videos. While the specifics are still unknown, it was announced generally that YouTube videos are now recommended based primarily on watch time rather than individual views. Watch time also ties into retention, which is also tracked. If a video is watched for only a few seconds, it isn’t enough to track a view and doesn’t push it higher in the recommendation algorithm. In addition, the percent of what a video is watched (regardless of its length) is also taken into consideration (Game Theory). Most amateur YouTubers consider an average retention over 50% for the entirety of their views to be ideal.
This shift also tied video views to the channel, rather than having individual videos count on their own. The shift was described as “a way to encourage creators to build up their channels, not just one single video” which was also indicative of the shift in content that YouTube was experiencing around that time (Bausch, Han; Game Theory). While LP and other forms of video content had been on the service since 2008, its long length and niche audience often led to lower numbers of views, resulting in decreased promotion through YouTube’s algorithms.
However, with the change focusing on compounded minutes watched across an entire channel, long-form content shifted into becoming more recommended than the usual short form. While previous videos had been focused on being short and re-watchable (comedy skits, vlogs, etc.) to farm clicks, now retention and longer videos that could keep user’s attention for longer
periods of time were more favored by the algorithms. This shift altered YouTube as platform from a medium designed for heavily edited, viral, shareable media clips that focused on quality and spreadability, to videos focused on retaining viewer’s interest through longer video content. This allowed for an otherwise niche video genre, the Let’s Play, to gain traction.
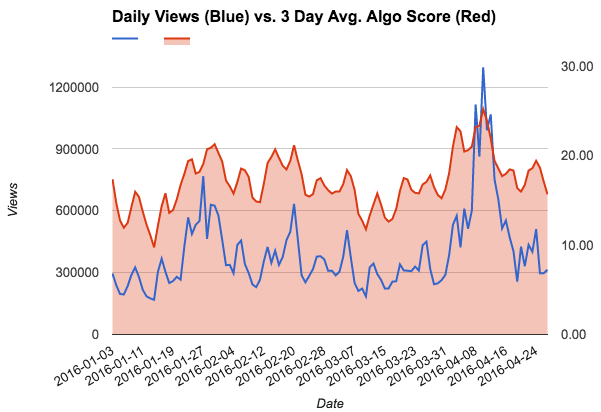
YouTube Algorithms