The first level of inquiry into the corpus, at the Tweet level, poorly accounts for the differences between pronouns data studied in this level, suggesting instead similarity in referent or inadequacy of methods. Variation at the level of tokens is examined in referent features (based on variables in previous corpus-based THEY studies) and in the presence of “minimal pair”-like
Tweets that show the target pronouns being used in nearly identical Tweets. These tests seem to indicate similarity in the tokens, rather than patterning with variation. Then, the strategy of measuring co-occurrence of pronouns with additional qualitative features of Tweets (ex. Retweet tags) is rejected, as it is found to poorly represent this data.
Lack of variation in referent
Previous authors focused on how features of the referent varied between THEY, SHE and HE; Tweets were exploratively but ultimately, uncompellingly tested for several of these features when separable from antecedent. Several of the previous studies reviewed above deal narrowly with the combination of pronouns and antecedents, distinguishing between, for example,
quantifiers (gender-marked or not), generic definites, existential indefinites, and (unattested with THEY) names and deixis in Lagunoff (1997). Though each author offers a slightly different taxonomy of possible antecedents, it is clear that none will account for the tokens included in this analysis, which, definitionally, are all linked to the same antecedent (#oomf). Furthermore, as established above, in the vast majority of Tweets, #oomf is a specific noun-phrase
antecedent – what Lagunoff (1997) and Newman (1992) each claim to be the least likely of the possible antecedents to be linked to THEY. Still, this assertion of unlikelihood poorly accounts for this naturally occurring corpus, in which THEY occurs at nearly the same number of times and SHE or HE.
Several features proposed independently of antecedents were tested on a small, random sample of Tweet texts to confirm that, in practice, these features did not compellingly correlate with pronoun variation within this corpus. I examine gender marking in the Tweets alongside several referent features analyzed in previous studies: notional number, referential solidity, and individuation. Notional number (Newman 1992, Baranowski 2002) allows for the possibility of multiple people as the eferent (for example, ‘Every #oomf tweeted about their day,’ if it were in the corpus, could be judged to be notionally plural). Referential solidity (Newman 1992) tries to capture the idea that a referent may or may not actually exist, such asin the fictional Tweet ‘I wish I had #oomf that would Tweet about his day’. Individuation, which also plays an important role in the observations of introspective studies like McConnell-Ginet 2011a, 2011b and Mackay 1980, is the concept that some people enter discourse as prototypes and not as specific characters; Newman (1998) quantifies it on a scale from 1 (least
individuated) to 5 (most individuated), but I find this degree of distinction untenable for such short texts and reduce it to 1-3. The concept of gender applied here is my own: attempting to recognize that looking for gender only in the pronoun would beg the question, I look at all other aspects of the sentence for gendering of the referent. I thereby recognize that gender is not a
salient feature outside of antecedents and pronouns in most sentences and finding THEY in sentences without additional gender information does not prove a unique point about THEY. In practice, I find gendering in my samples with words like “bro” and “bitch” though I looked in principle for any overtly gendered NPs, modifiers, or predicates associated with #oomf. A table
defining these measures and tables showing them applied to SHE, HE, and THEY are included in the four tables of the appendix. This small, random sample serves to illustrate that the features associated with THEY in
other studies neither apply well to THEY in these cases, nor do they vary between the pronoun groups in this corpus. Each pronoun group had other gender information in only one of ten Tweets. The #oomf in every token was plausibly singular and solid, referring often to past and ongoing interactions with specific people or to physical appearance. Because of this level of
specificity, all samples except one THEY Tweet are judged to be fully individuated. It seems that all these traits approached the idea of ‘specificity’ of the referent but that in this corpus, which, with this antecedent, shows little difference across pronoun groups. As previously examined aspects of the referent fail to motivate distinctions between THEY, SHE and HE, this small
sample provides strong evidence that the salient and compelling distinctions between the target pronouns in this corpus are to be found elsewhere.
Cross-pronoun Tweets
Indeed, the similarities between pronoun-including Tweets extend beyond conceptualizations of the referent, but are written also into the entire Tweet texts. A close examination of the pronoun subcorpora reveals that several Tweets cross between corpora. While some of these Tweets seem to be derivations of each other, others seem to be rather mundane messages that
were simply expressed by several users, linking different pronouns to #oomf. Inconsistencies among similar messages’ typography (ex spelling, punctuation) and sentence structure are strong evidence for or against shared origins (or at least a desire to display shared origins) in this context, where messages are so easily and so often copied and pasted. In the following, several sets of Tweets illustrate that THEY can alternate with SHE or HE both incidentally and,apparently, through intentional replacement.These Tweets are something of minimal pairs between the pronouns, supporting the hypothesis that it is the pronouns themselves that contrast with each other in the sentence and suggesting that attention be paid to the context rather than the sentence. A sentence with SH is not distinguished from a sentence with HE by the propositional content of the sentence, but rather by the appropriateness of the reference in the context (cf Heim and Kratzer 1998). For example, between in the following two pairs of Tweets, the contrast would be described in terms of felicity conditions: the sentence is meaningful if and only if the features of the pronoun, to begin with, that of gender, match those of the referent. Linguists, when describing these, would not look to the minor differences between them (like the replacement of “does” and “if”) to explain this difference.■ I Wonder Does #Oomf Ever Think About Me Like I Think About Him..?
■ I Wonder If #Oomf Thinks About Me, Like I Think About Her?Similar pairs are found between THEY and HE, once again with no propositional content of the sentence offering clues about what distinguishes the two pronouns from each other.
These especially question how the features of the referent that were examined above in random tokens could be applied on a token level: if THEY does imply a less individuated or notionally plural referent, that would not be discernable from these sentences without begging the question.
■ hoping #oomf sees all these tweets about them….
■ I hope #oomf sees my last tweet and knows its about him.
And:
■ #oomf going through. Poor baby. He needs a hug.. ■ #oomf going through hope they gone be alright
[Note: ‘going through’ means roughly ‘going through a difficult time’] One particular predicate is used with #oomf in Tweets about SHE, HE, and THEY. These Tweets assert that #oomf “could get it,” meaning “could have sex with somebody” (usually
meaning the speaker, where not further specified). This is a common comment about #oomf in the corpus (including in several Tweets that did not include target pronouns). Apparently, a pronoun must not encode information about the referent’s gender to be used with this sexual predicate. In the following, “can get it” is used with all three pronoun groups, in a and b with
THEY, in c with HE, and in d-f with SHE.
a) Yeeeeessss!! RT “@USER_A: #oomf just don’t know how bad they could get it” b) #oomf just don’t know how bad they could get it c) RT @USER_B: If #oomf hadn’t fkd my friend he could get it something in context, rather than within sentences, conditions the appropriateness of the pronoun used.
Token-level co-occurrence
Based on the methodology of the Twitter literature cited in the above sections, an attempt was made to annotate and cross-tabulate additional features of Tweets, such as the co-occurrence of the target pronouns, Twitter tags (@-mentions, Retweets, additional hashtags) and additional pronouns. This was done using a similar Python script to the one that found third-person pronouns, although these features were generally more straightforward and did not require human input. Ultimately, these results are not included in detail here because these co-occurrences were greatly skewed by a certain tendencies of the data at the token level, and the subcorpora were inconsistently reduced at the type level. For instance, the most compelling among mostly-insignificant measures was the finding that fewer of the Tweets including SHE or HE were marked as Retweets (about 32% and 25% of each subcorpus, respectively) than the corpus in general (about 54%), while a larger share of THEY Tweets (64%) were Retweets. These correlations could be read similarly to the results that motivated, among .
others, Naveed et al’s (2010), Suh et al’s (2010) and Yang et al’s (2010) accounts of what makes a Tweet more retweetable. However, to me, it seems too strong a claim to make that a pronoun sparks retweeting behaviors or even to suggest more moderately statements about the sharability of the Tweets’ content. Rather, based on closer examination of the subcorpora,
these likelihoods of retweeting were determined by a few aberrant cases, while most THEY Tweets were spread quite similarly to the Tweets in the other subcorpora. That is, upon closer examination of these subcorpora, it did not seem to be the case that each Tweet enjoyed equal chances at interaction, by virtue of containing a certain pronoun. Instead, the pattern emerged
on a corpus level once connections between heavily retweeted texts were established.
Sub-corpus composition
The following section introduces another method of analysis of this corpus: clustering Tweets based on shared texts at their cores. In what follows, I ground my method of clustering Tweets around what I call “cores” (recognizably shared texts) and then describe the results of this process, which reveal a very different composition of the THEY subcorpus than of the other
subcorpora.
Core texts” and clustering
Pronouns are features of individual texts, and Tweets the basic unit of interaction in Twitter; however, textual and social connections are performed within those texts and nuanced view of the linguistics of Twitter demands also an ethnographic eye to those connections and affiliations. Though the subcorpus of target-pronoun-including Tweets studied here contains
1139 unique Tweets and unique points of social action, those 1139 do not represent as unconnected, independent data points. In the following sections, I reject a view of this corpus as a two-dimensional stream of texts and instead associate the Tweets based on textual similarities.
The most basic and pervasive connection in this hashtag corpus is the act of reproducing another user’s text. Here, I look for more than paraphrase or shared sentiment; instead, I focus on clearly related Tweets that share a core text: what was apparently originally a bare Tweet and is now central to one or more Tweets, perhaps surrounded by Retweet markings and
additional commentary. This goes further than reducing the corpus from tokens to “types” based on string identity of the Tweet texts: such a narrow definition of sameness captures some connections but misses some of the most basic, finding, for example, the two iterations by separate users, with unique timestamps, of “’RT @USER: I Love #oomf And I Told Her” but
not the original version that is also present in the corpus, “I Love #oomf and I Told Her”. Instead,by clustering texts that apparently arise as the same “core” text is modified and appended, connected texts are brought together. Where certain clusters did not have a discoverable, “core” Tweet without any Retweet markings and the earliest timestamp, one could be inferred.This method exposes the intertextual and interpersonal connections written into the
corpus, but is closely tied to the time of sampling and is certainly a situated, partial account of connections made with the texts studied. For example, several Tweets that did not seem related to any other Tweets in the corpus were, nevertheless, marked as Retweets. This may be due to the limited timeframe of my sampling of the API or may reflect incomplete datasets. It is
impossible to know, also, which Tweets will later be retweeted or otherwise shared, which were not broadly shared at the time of collection. This points, more than anything, to the incomplete nature of my study and reflects a general principle of social science: that any study is necessarily a partial view of any entity from the perspective of a unique situation in time as
space (cf Bucholtz and Hall 2005’s “partialness principle”). Still, the emergence of interaction and scales of interaction in such a limited dataset does seem meaningful.My decision to study Tweets as “clusters”4 is grounded in the nature of the data as texts. Other authors, borrowing from computer science literature, refer to a “cascade” model of Tweets, using the term to describe how Tweets spread from user to user, being further retweeted from each node (referred to in, for example, Cha et al’s description of influential users setting off ‘cascades’, 2010 p 2). This does more accurately capture the networked
behavior behind Tweeting dissemination. In this paper, however, I avoid the term because my data is not nuanced enough to reliably reveal cascades. Twitter texts do not encode connections between users or texts, except those made explicit in texts through @-tagging or Retweet tags. In this way, much of the networked behavior between Tweets is neither visible to
the researcher, nor to users who did not immediately take part in it. Where native Retweets arretweeted from an intermediary, rather than the original author, they are still only attributed to the original author; user-typed Retweet practices, in turn, are inconsistent in this regard. Furthermore, some clusters emerge without full or accurate attribution to an “original” source,
but, nevertheless, consist of non-trivially similar texts; these seem to participate in the spread of the text while perhaps remaining ambivalent about user-to-user connections. Therefore, I ground this analysis in the data itself, which offers rich intertextual, if not interpersonal, information. By this I mean, patterns and trends are observable between texts, beyond those
present in individual texts, and I now turn my analysis to those.The corpus was clustered semi-automatically with a Python script and my input. A Python script was passed through each pronoun group subcorpus, and the combination of all
pronoun-including Tweets to find close textual matches based on substring insertion and deletion. This definition of closeness offers the advantage of reflecting how Tweets move between users in Twitter: by attaching textual connections to other users (e.g. Retweets), using copy-paste, and insertion and deletion (which leaves intact anomalies like idiosyncratic capitalization), rather than making character-level changes. The clusters were then reviewed to ensure that they seem to constitute a meaningful connection between texts; this is illustrated in the following two examples, one of texts that are clearly derived from a common source, and another that shows incidental similarities. Clusters that did not seem to reflect purposeful
reproduction of other messages were split.The following illustrates a set of Tweets clustered based on a common core Tweet (a) where the Tweets show diverging and layered Retweet practices (see differing Retweet techniques of b, which marks the Retweet with RT, and c, which puts the attribution and text in quotation marks; d is another layer of Retweet over c, where a string insertion at the end of cthat is reproduced in d (“RTF” for “re-fucking-tweet”). (Note: “hitting up” a person means
“initiating communication with.”)a) I’ll stop hitting #oomf up now. For good. I won’t put the effort in if they won’t.
b) RT @USER_A: I’ll stop hitting #oomf up now. For good. I won’t put the effort in if they won’t.c) “@USER_A: I’ll stop hitting #oomf up now. For good. I won’t put the effort in if they won’t.” RFTd) RT @USER_B: “@USER_A: I’ll stop hitting #oomf up now. For good. I won’t put the effort in if they won’t.” RFT
Clusters were manually split where they did not seem to capture common origins of the Tweets. The following Tweets, for example, seem to be clustered based on common form of, “I wanna ____ #oomf but ____ don[‘]t wanna ____ them … ”. However, this message does not seem to produce particularly unlikely sentences or bring together unlikely typography, other RT connections were apparently made between smaller subgroups, and the meaning of the Tweets connections were apparently made between smaller subgroups, and the meaning of the Tweets in relation to each other does not suggest that the users were trying to connect them. Therefore, I determined these Tweets to actually represent two sets of clustered Tweets (a-c, d and e) and one independent Tweet (f). The hyphens and underscores at the end of 6 constitute a bored or neutral faced emoticon.a) I wanna save #oomf but they dont wanna save themself <<<b) “@USER_A: I wanna save #oomf but they dont wanna save themself <<<”c) RT @USER_A: I wanna save #oomf but they dont wanna save themself <<<d) I wanna text #oomf but I don’t wanna text them first. I would feel awkward.e) RT @USER_D: I wanna text #oomf but I don’t wanna text them first. I would feel awkward.f) I wanna talk to #Oomf but I don’t wanna bother them -___-This method of analysis does not suggest an increased tendency to retweet any given Tweet based on features; rather, it exposes the emergence of several large-scale THEY Tweets while all other Tweets bring together the interaction of only a few or one users. This perspective on the corpus uncovers a clear difference in the composition of the THEY subcorpus.b. Cluster patternsWhile each pronoun subcorpus showed many Tweets on a small scale, passing mostly through only one or two accounts, the THEY corpus included fewer cores in total, and it was nearly half comprised by only two core texts. These were Tweeted 102 and 76 times.The breakdown of the number of unique cores is compared to the number of tokens, or unique Tweets, in Figure 3 below. This graph illustrates that, although each pronoun group occurs in approximately the same number of Tweets in the corpus, there are many fewer messages that make up the THEY Tweets (149 cores) than SHE and HE. SHE (310 cores) and HE (290 cores) remain somewhat comparable, however, with roughly twice as many cores.
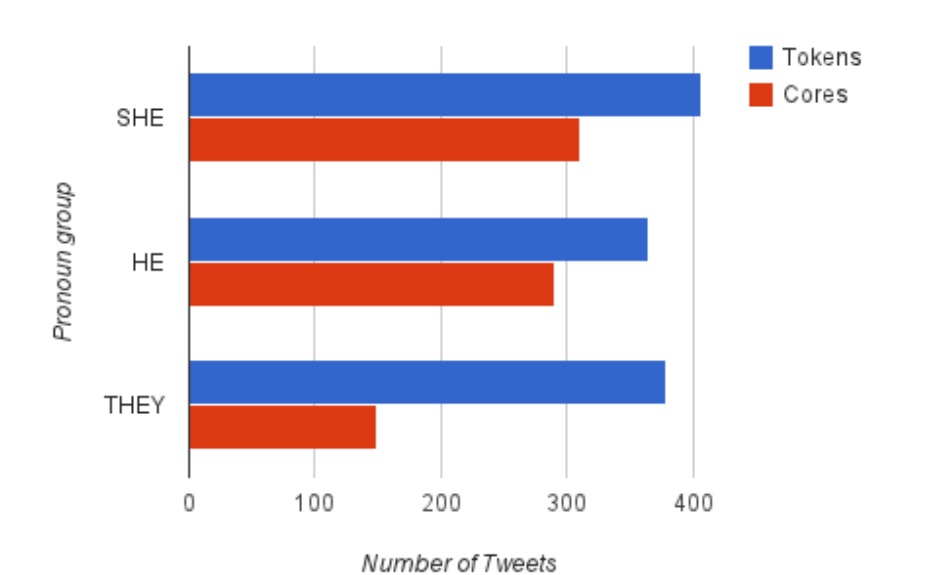
Number of Tokens (unique Tweets) and number of cores (unique core texts) by pronoun
group.
However, it is the scale of several of the clusters that most compellingly distinguishes the THEY subcorpus from the others. All clusters of five or more Tweets are shown in Figure 4 below; this is five clusters each for SHE and HE and six for THEY. But while the largest SHE and HE clusters represent maximally 8 and 14 Tweets, respectively, two THEY clusters reach a
different scale of popularity, with 102 and 76 unique Tweets constituting each cluster by virtue of being built around the same core.

All clusters of 5 or more Tweets are shown
Figure 7 (THEY) below. The figures show how each pronoun is tweeted with different levels of uniqueness. Clusters of four or more Tweets are represented individually, while smaller cluster are aggregated into one slice (for example, the “triples” slice in Figure 6, showing clusters in the HE subcorpus, represents five clusters of three Tweets each, and is weighted to take the space of fifteen Tweets). While the THEY subcorpus is dominated by the two large clusters, single- and two-Tweet clusters dominate the SHE and HE subcorpus. Still in each, only about ten to twelve percent of clusters reach a small-intermediate size: even THEY has a strong showing of cores that do not move between users.Figures 5 and 6, illustrating the makeup and SHE and HE respectively, show very similar make-ups. For SHE, 63.4% of the subcorpus is made up of “singles”, or clusters made up of a core that is tweeted only once. An additional 13.8% is contained in clusters of two Tweets, and 10.3% in clusters of three Tweets. The remaining 12.5% are in 10 clusters of four to eight Tweets each. Tweets in the HE corpus were disseminated on a similar scale. 69.5% of that subcorpus is made up of “singles.” 14.3% of Tweets are part of two-Tweet clusters, and 4.1% part of three-Tweet clusters. The remaining 12.1% of the corpus is included in six clusters of
four to fourteen Tweets each.For THEY, however, smaller-scale participation exists alongside massively public Tweets.
31.7% of the subcorpus is made up of cores that occur only once, 10.0% of two-Tweet clusters, and 1.6% of three-Tweet clusters. Six small clusters of between 4 and 10 Tweets up 9.7% of Tweets in the subcorpus. Finally, only two cores constitute the remaining 47% of Tweets including THEY, as they are passed from user to user: A 76-Tweet cluster represents 20.1% of
the corpus and a 102-Tweet cluster, an additional 26.9%.