Tactical operating room planning and scheduling
One strategy for cost containment in activities surrounding the operating room (OR) is effcient utilization of OR resources through scheduling optimization. As OR-related costs contribute, on average, 8-10 percent of a hospital’s total expenses, OR scheduling optimization is an extremely important task. Furthermore, inefecient use of ORs through mismanagement of available OR time and surrounding OR resources can lead not only to increased costs, but also to prolonged patient wait times surgical case cancellations, and overall patient dissatisfaction.
OR planning and scheduling across multiple ORs has been widely studied in the literature. Two main tactical planning strategies are commonly used: block scheduling and open scheduling. In block scheduling, all available OR time is divided into discrete time intervals, called blocks. Surgeons, or groups of surgeons, are allocated to each block, and each group schedules patients freely within the assigned block. In contrast, in open scheduling, surgeons are not scheduled to work within blocks of time, but instead perform surgeries whenever they are available and the appropriate hospital resources are free. In this paper, we focus on assumptions commonly made in data generated for open scheduling type problems. However, many of these assumptions are also made in block scheduling and some of our conclusions are applicable across planning strategies.
In general, each paper in open scheduling optimization chooses a different set of data on which to run experiments, and there is no standard data set that is frequently used. To generate data, researchers will attempt to sample from real data if it is available, but will randomly generate any data that is missing as realistically as possible. Unfortunately,
there are at least two potential problems with the current data generation practice in the literature. Firstly, since researchers are working with different data sets, it is difficult to assess computational results across different algorithms in the literature. Secondly, it is challenging to assess the integrity of the models as compared to real scheduling situations found in hospitals. In general, assumptions made in the data generation process can impact the conclusions made in any single test. In this paper, we identify some important decisions commonly made in the data generation process, and seek to quantify the impact of these decisions. We will shed some light on which assumptions in the literature are justied and which elements should be highlighted and reworked.
Many different types of data are needed to simulate a typical hospital. Cost-based objective functions are commonplace in the open scheduling literature, necessitating data regarding OR and surgeon-related expenses. This information is often unavailable to researchers, subjective, and subject to change. As a result, although these numbers sometimes originate from consultations with OR scheduling decision-makers, many papers choose not to delve too deeply into accurate costing, instead determining costs based on averages determined by past studies. Various surgery-related durations such as surgical time, OR cleaning time, and OR preparation time for each operation are also needed for OR scheduling models. In the OR planning and scheduling literature, researchers have sampled directly from real data, or have simulated data based on uniform, lognormal, normal or Pearson III distributions. In the OR simulation literature, the lognormal distribution has been used. Although there is no consensus on the correct distribution to use, the lognormal distribution has been shown to be a reasonable choice to simulate surgical times in at least one study.
In addition to surgical durations, any data generation scheme must decide on allowable patient-resource assignments; in particular, allowable patient-OR and patient-surgeon assignments must be determined. Surgical cases are often considered to be assigned to a single surgeon and able to be operated on in any room; however, general models that include opportunity for partial patient-surgeon and partial patient-room exibility have been proposed, and accurately represent realities within some hospitals. Data sets must also consider realistic resource availability schedules. Almost always, ORs are allowed to be open for 8 hours each day, whereas surgeons are available on certain days of the week on a rotating schedule.
Since we are working in tactical OR planning, we deal with a rotating schedule based on a week-long planning horizon. Some patients must be scheduled within the current planning horizon, others can be pushed to the next planning horizon. If data is not available on the percentage of patients that must be scheduled in the current planning horizon, this data must also be estimated. In our case, it is set at 50%, assuming half of the patients should be scheduled during this planning horizon. Furthermore, each patient of this planning horizon should have a deadline before which patients must be scheduled. One important implication of this hard deadline is that a naively generated data set is not necessarily feasible, and any data generation scheme must ensure that there is at least a single feasible schedule. It is not obvious how many papers in the literature ensure schedule feasibility.
In general, data generation is not simple, and there are many assumptions that must be made to create reasonable input data for our models. Thus, it is unsurprising that the data sets used in the literature vary widely. Our research attempts to assess the impact of using dierent data generation methodologies.
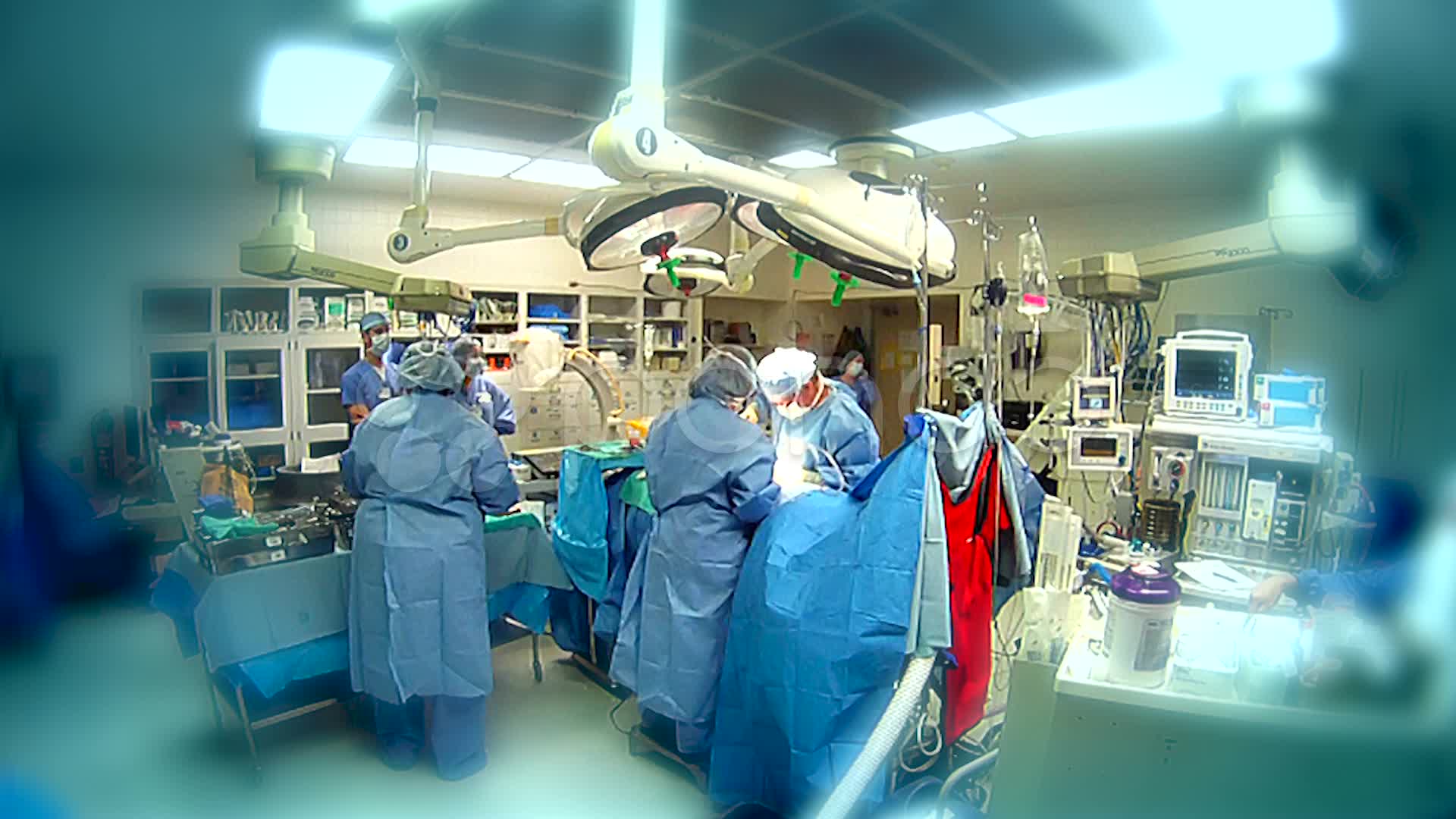
Tactical operating roomTactical operating room