Resilient Systems
- AI obstacle
Two issues with advancing AI usage are the lack of extensive knowledge base capabilities, and the ability to learn. The element of complexity appears to be that an AI system must retain a vast spectrum of knowledge and traits that allow it to write programs for specific conditions it cannot answer. AI experts note that problems with artificial intelligence and involved programming technology are so complex that algorithms and standard programming methods are insufficient to solve them. Although this argument bares truth, it does not detail the advances in AI. Other opponents or arguments against AI have noted problems pertaining to memory capacity and order. Advance knowledge of information storage requirements and memory organization infers that programs need flexibility. These perceptions introduce a state of stagnation with AI. Artificial Intelligence (AI) advancement made ground early on but has had less concentration and research because of the impacts of these observations and the belief in the condition of system restriction.
Resilient control systems
Resolving the current enigma within Critical Infrastructure
(CI) depends on resilient designs. Designs of current systems depend on operator reprogramming and or repair after the fact. By designing systems that consider all threats and measures, the problems confronted in CI can be alleviated. The dated definition of resilience fails to consider the current state of security for CI. The ideology of organizational and information technology in association with resilient systems are problematic. A terminology that suggests systems can tolerate fluctuations to their structure and parameters fails to account for malicious deeds . One alternative is the idea of Known Secure Sensor Measurement (KSSM). “The main hypothesis of the KSSM concept is the idea that a small subset of sensor measurements, which are known to be secure , has the potential to significantly improve the observation of adversarial process manipulation due to cyber-attack” . Resilient systems should be able to determine uncertainties, sense inaccuracies under all conditions, take preventive action, recover from failures, and mitigate incident beyond design constraints. Valid resilience considers representations of proper operation within process applications when facing varying conditions inclusive of malicious actors and includes state awareness within the resilient design.
Cyber awareness
Awareness in the cyber domain intended governing to happen through
risk assessment. Only forensic evaluation after the fact truly indicates the actual cause of an abnormal event. Predictability in determining critical digital assets is difficult to impossible in regard of hidden dependencies . The intellectual aptitudes of malicious actors improve through the usage of stochastic methods whereby variability of motive and objective exist . Put simply, risk management allows no technical review of potential risk and is really a business tool . A huge misnomer resides in the condition that risk mitigation will allow defensive metric implementation in ample time. Cross-reference this with CI and the idea is flawed. Rapid reconfiguration in these environments is not a possibility; due to their design, the probability of mitigation is near impossible . Though routine and common pattern analysis may provide anomaly comparisons, its limitations in predicting an adversary’s behavior is minimally effective. The three principles provided in Table 2 should be the basis for policy and the way forward.
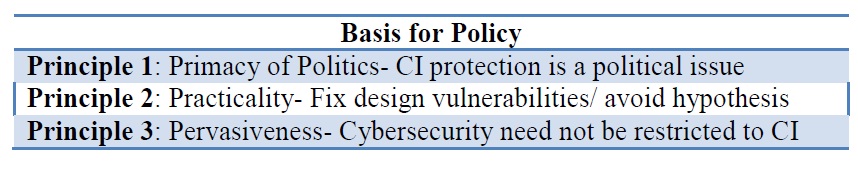
Table 2
Basis for Policy
Viewing CI from as a political issue is precedent. Fixing design vulnerabilities should be paramount and should not include hypothetical solutions or assessments. Though CI is vital the security of the cyber domain should be viewed unilaterally.