Few areas of image processing have the kind of impact that medical image processing does. From performing reconstructions from MRI and CT scans to contrast enhancement of X-rays to techniques aimed at allowing more automated diagnoses by physicians, advancements in medical image processing have the potential to save lives and to save medical facilities time and money by realizing improved efficiency in delivery of care.
One area of particular difficulty is the process of segmenting blood vessels from medical images. As a brief review, the human vascular system is composed of arteries which carry oxygenated blood from the heart out the the extremities; capillaries, where gases and nutrients are exchanged; and veins, which carry deoxygenated blood back to the heart and lungs.
Imaging of the vasculature is carried out for many reasons: to detect blood clots in veins, to diagnose stenosis (narrowing) of arteries, to monitor cerebral vessels for postoperative vasospasm, and for early detection of atheroscelrosis (plaque build up on the walls of the arteries), and to support many other diagnoses. These imaging studies are carried out using a wide-variety of imaging modalities as well: ultrasound, fluoroscopy, computed tomography (CT) angiography (using X-rays), magnetic resonance (MR) angiography, and in rare cases even visible light can be used. In particular that is the case with retinal fundus imaging, the application area of this work.
Many typical approaches to segmentation rely on texture information or the fact that objects of interest (like cars and people) form closed contours in images. This is not the case with blood vessels. Blood vessels do not necessarily follow simple paths, but instead can be “tortuous” as clinicians describe them. In addition the size (or caliber) of vessels varies tremendously, from millimeter wide capillaries to the 300mm aorta. The changes in size serve an important physiological purpose: to maintain sufficient blood pressure to perfuse the tissues in the body, but they make the task of automatically finding vessels difficult.
Our particular interests are, long-term, in the cerebro-vasculature (the brain’s blood supply), and more immediately (in this work) segmenting blood vessels from retinal fundus images. Retinal fundus photography uses visible light to image the interior of the eye, allowing the physician to inspect the vasculature, the optic disc, and the retina. There are also several kinds of lesions that a physician might be interested
such as hard exudates (See Figure 1) which are lipids deposited in the eye from leaking capillaries. The patient in this image most likely has diabetes and/or hypertension (high blood pressure) We are not focused on detecting these other structures in this work, but they do provide additional challenges for our vessel segmentation procedure because along with the blood vessels they are also “salient” objects that we must not confuse with vessels.
Compared with other imaging modalities retinal fundus images have readily available benchmark data to enable us to validate the direction of our research before moving on to more challenging types of images such as computed tomography (CT) angiograms of the cerebro-vasculature.
Retinal fundus images are essentially visible light photographs of the eye taken through a microscope lens. As a result they present several challenges to effectively analyze (refer to Figure 1 again as it exhibits nearly all of these problems)
1. Aperture effects from the lens (the sharp circular border at the edge of the eye pixels)
2. Uneven illumination from the light source on the camera
3. Pathology (hard exudates, red lesions, etc.)
4. Extravascular structures (the dark macula in the center of the image, the bright optic disc).
5. The irregular structure of the vessels (for example, try to image the convex hull that contains all of the vessel pixels in Figure 1)
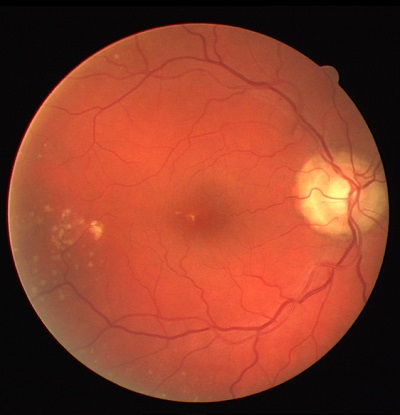
Figure 1: Challenging DRIVE image showing vignetting from poor lighting, the presence of exudates (the yellow spots), and central vessel reflex.